본 포스팅은 고려대학교 산업경영공학부 강필성 교수님의 [Korea University] Business Analytics (Graduate, IME654) 강의 중 04-7: Ensemble Learning - XGBoost 영상을 보고 정리한 내용입니다.
1. XGBoost 개요
- 어떻게 하면 original Gradient Boosting보다 빠르고, 더 많은 용량을 처리할 수 있을까?
- 근사 기법을 적용함에 따라 GBM에 비해 약간의 성능 저하가 있을 수 있지만, 더 빠르게, 더 많은 데이터를 처리할 수 있음, 병렬 처리도 가능함
2. Split Finding Algorithm
- Exact greedy algorithm :
+ 모든 가능한 split point를 탐색하기 때문에, 언제나 optimal한 split point를 찾을 수 있지만, data가 메모리에 다 들어가지 않는 경우 적용이 불가하며, 분산 환경에서 처리가 불가능함
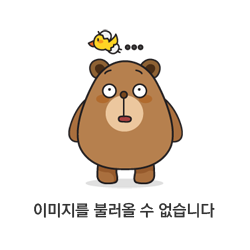
- Approximate algorithm :
+ 각각의 변수(k)의 데이터를 l개의 bucket으로 구분하여, bucket별로 split point를 탐색, 보통
+ split의 candidates 수도 적게 가져가면서 bucket에 대해 병렬 처리 가능
+ exact greedy algorithm에서 도출되는 split point를 찾지 못할 수도 있음
+ bucket을 tree별로 구분할 수도 있고 (global variant), bucket을 split별로 구분할 수도 있음(local variant)
+ local variant보다 global variant의
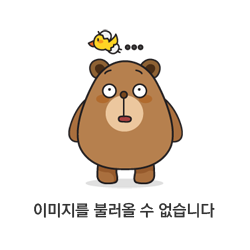
- Exact greedy algorithm 과 Approximate algorithm 비교
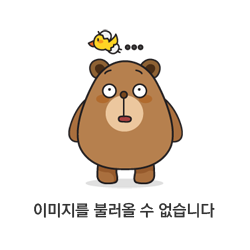
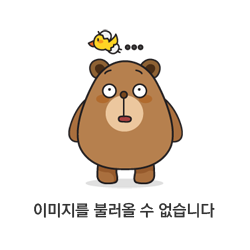
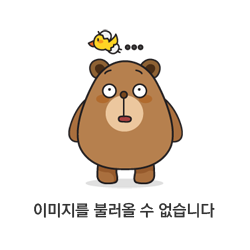
3. Sparsity-Aware Split Finding Algorithm
- 현실 데이터는 sparse한 경우가 많음, missing value가 존재하거나, 값이 0인 데이터가 많음 (categorical data에 대해 one-hot encoding을 수행해서 이러한 경우가 발생하기도 함)
- split마다 default direction을 정의하여 새로운 데이터에 missing/0 데이터가 존재할 때, default direction으로 보내버림(분류함)
- sparsity-aware algorithm은 basic algorithm에 비해 계산 속도가 빠름
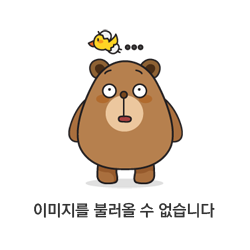
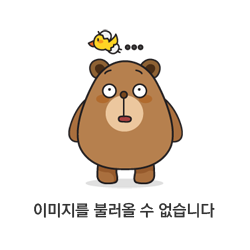
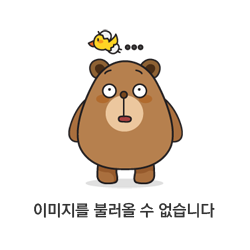
4. System Design for Efficient Computing
- 데이터 저장 형태 :
+ 각 변수의 sorting 과정이 tree 학습 시 시간을 가장 많이 할애하는 부분
+ XGBoost에서는 미리 sorting해서, column-wise 형태로 데이터를 저장하여, tree 학습 중간 중간마다 sorting할 필요 없음
- Cache-aware access
- Out-of-core computing
- Block Compression
- Block Sharding
참고 자료
- T Chen, C Guestrin (2016) XGBoost: A Scalable Tree Boosting System, KDD '16: Proceedings of the 22nd ACM SIGKDD International Conference on Knowledge Discovery and Data Mining, August 2016 Pages 785–79
'머신러닝' 카테고리의 다른 글
[분석 방법론] Ensemble Learning(8) - LightGBM (0) | 2023.01.01 |
---|---|
[분석 방법론] Ensemble Learning(6) - Gradient Boosting Machine(GBM) (0) | 2022.12.28 |
[분석 방법론] Ensemble Learning(5) - Adaptive Boosting(AdaBoost) (0) | 2022.12.12 |
[분석 방법론] Ensemble Learning(4) - Random Forests (0) | 2022.12.12 |
[분석 방법론] Ensemble Learning(3) - Bagging (0) | 2022.11.29 |
댓글